Revolutionizing Consumer Insights Through Text Analytics
Organizations are striving to enhance their capabilities in listening to customer feedback effectively and swiftly translating insights to actions This is readily apparent through investments made in building a robust insights ecosystem.
Customer surveys remain one of the key elements of an insights ecosystem. A short, 2 to 3-minute survey asked of customers who may have recently visited a store or made an online purchase typically consists of between 8 and 10 scale-based or multiple-choice questions and a couple of open-ended questions.
Whilst the scale-based questions get analyzed in detail and are used for tracking performance across time or across segments, benchmarking, arriving at staff KPIs, etc the question we need to ask is, are we doing justice to the open-ended responses?
Open-ended feedback is a rich source of insights that are often not utilized to their full potential due to the complexity involved in this data and quantifying its impact on the KPI. Thus, it’s not a surprise that well-established experience management platform providers offer text analytics as a part of their higher suite of offerings.
Why invest in understanding open ended feedback?
Big picture vs the BIGGER PICTURE
Just relying on scale-based rating questions to capture the experiences of customers with an organization has limitations as your customers would like to give you feedback on a million aspects and it’s not feasible to have a scale-based question for each of these aspects.
Advanced text analytics (ATA) platforms have the built-in capability to classify customer feedback into hundreds or thousands of topics to give organizations a complete and comprehensive view of customer feedback.
Understanding what impacts the KPI
Though your scale-based questions can help you understand the movement in your KPI (NPS, OSAT, etc) to some extent one cannot afford to ignore the additional information present in the open-ended questions
Advanced text analytics can help you understand the holistic picture beyond ‘scores’ and can accurately help pinpoint the reasons for the movement in the KPI as well. Yes, results from the open-ended feedback can be quantified and linked to the KPI to understand what impacts and drives customer experience.
Being proactive rather than reactive
The entire cycle of customers giving feedback and the feedback getting reported to the relevant stakeholders can take some time, and in the interim period, certain critical issues can blow out of proportion and impact a larger set of customers.
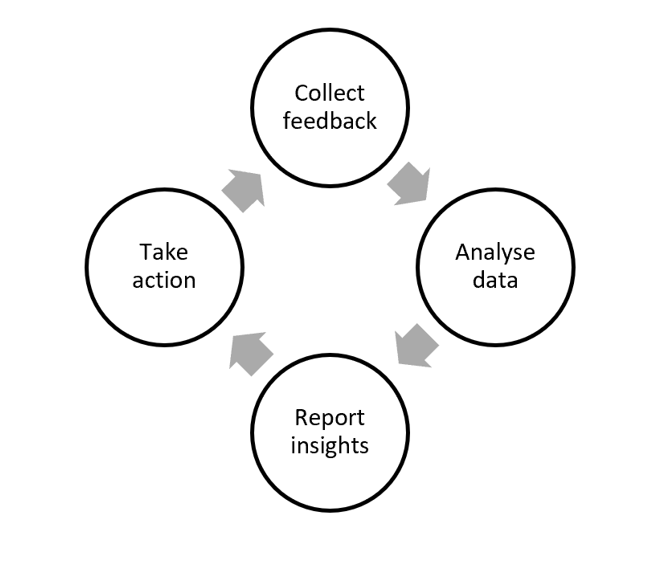
Once a well-designed analysis has been set up on an advanced text analytics platform it can make this process more effective and efficient. An organization can also gauge early signals of budding issues before they explode into bigger drivers of dissatisfaction.
Extracting relevant insights for different stakeholders
Different departments within an organization need to work in sync like a well-oiled machine to deliver an optimum customer experience and it’s important that they have quick & easy access to relevant customer feedback to make this possible. For example, capturing the experiences of customers for an online purchase would involve the website design team, product team, operations & logistics team, possibly customer service team, etc.
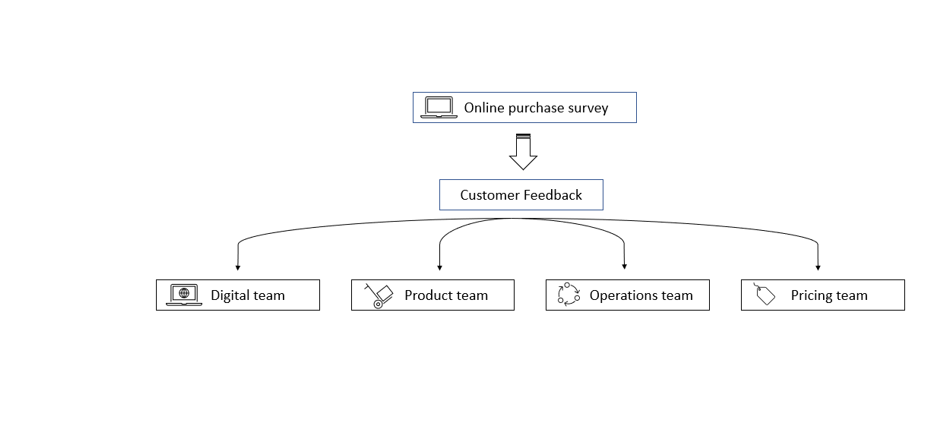
Advanced text analytics platforms make it possible to set up customized reporting for different stakeholders by only including elements from customer feedback that is relevant to their scope of work. Thus, one can cut through the noise and be super focused on what matters the most.
Avoiding lengthy surveys
When designing surveys CX professionals strive to strike the right balance between the number of questions asked and the survey length as a long survey can impact response quality and increase dropout rates whereas a very short survey may possibly not answer all questions from different stakeholders
Advanced text analytics allows CX professionals to design shorter and sharper surveys by typically including the KPI and open-ended questions as the core questions. This approach helps organizations focus on understanding feedback that customers would like to share rather than forcing customers to answer questions the organization is interested in knowing. Limiting survey length and utilizing advanced text analytics to understand open-ended feedback on numerous topics is likely to be the future of CX surveys.
Avoiding the trap of making frequent questionnaire changes
Often, there are times one would like to understand the views of customers on new initiatives introduced or gauge the impact of any seasonal aspects or temporary external factors, and it may often be tempting to add a few questions to the survey
One can keep a close eye on these things using advanced text analytics without making any amendments to your existing survey as customers are likely to talk about things that impact their experience in the open-ended question.
Linking customer feedback to customer journeys
Organizations are increasingly aiming at understanding customer experience through the lens of different journey stages they typically pass through in order to identify and address the pain points customers encounter at each stage.
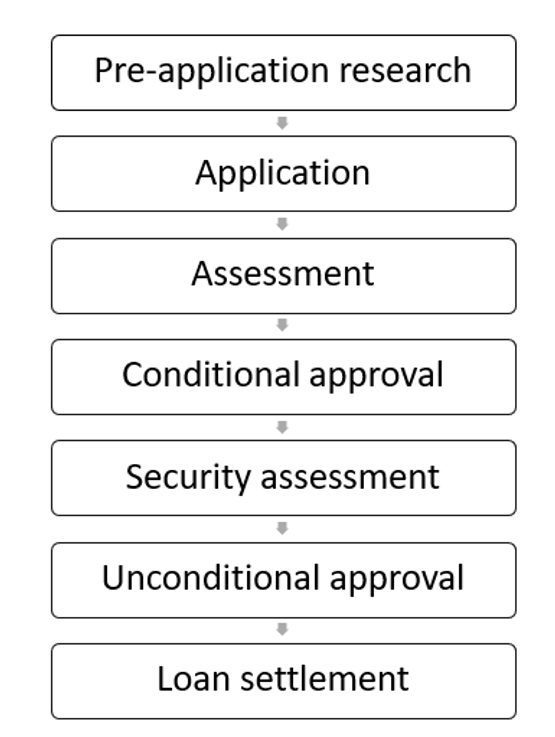
It may not be feasible to send out surveys after each journey stage, but advanced text analytics platforms can classify customer feedback and attribute it to different journey stages. Example – If a bank sends out a survey to a new home loan customer who has recently been through the application process, advanced text analytics platforms can classify and attribute feedback to different journey stages to allow clarity on customer experience at each stage.
Reducing the need for avoidable follow-up research activities
At times, though research projects bring to the surface certain issues at a very broad level, organizations need to invest in a more focused follow-up research activity to better understand certain areas of customer feedback in greater detail.
It is likely that customers have touched upon those areas in their open-ended feedback and that feedback can be systematically understood in detail through text analytics.
Advantages over traditional coding
Researchers have relied on coders for a long time, and it has worked well as coders go through every comment and classify them into different topics as appropriate. However, today advanced text analytics platforms offer researchers a much more powerful alternative that allows them to achieve above and beyond what coded results can.
In the case of large datasets, coding can be time-consuming as its manual.
- Coding can possibly end up being a costly affair if done frequently for large datasets.
- There is an element of subjectivity involved as rules may not be clearly defined.
- It can’t be applied to historical data, unlike advanced text analytics platforms where it’s possible at a click.
- Ability to interact with coded data is minimal unless the data is being imported into an analytics platform.
- In manual coding, there will be a limit to the number of topics that can be created, whereas, in advanced text analytics platforms, there can be hundreds or thousands of pre-created topics.
The 5 Key parameters for selecting the right text analytics platform
Text analytics can produce magical results but selecting the right text analytics solution could be a daunting task for those who haven’t had much prior exposure in this space.
Here are a few key questions one needs to ask while evaluating the right solution.
1. Setting up topics
This is one of the most critical aspects of the text analytics process, and it lays the foundation of a robust platform. You need to investigate if the onus of setting up topics is on the provider or on the user or is it hybrid.
Creating the right topics goes above and beyond entering just the keywords and it requires the right training, skillset, and effort in case you need to make a start from scratch. There could be n number of ways to say the staff was not helpful or the internet connection was not up to mark.
It’s easier for the provider to set up the topics for the user as they have the necessary skills and trained resources at their disposal to do so and importantly, they would have access to a lot of databases during their R&D to build robust topic rules. This will allow the user to hit the ground running and start using the insights quickly.
However, the users will need to give their input or advise the provider about organization-specific elements that need to be incorporated into the structure.
Thus, the recommended approach would be collaborative in nature with the provider taking the lead initially and training the users in the process.
2. The granularity of topics
The granularity of topics has a direct bearing on the effectiveness and actionability of the results. The more granular the topics are, the more detailed the insights. For example; - Staff behavior is a broad topic, but topics like staff friendliness, staff professionalism, staff efficiency, and problem resolution are sharper topics.
An effective platform should also allow the user to group specific topics into larger topics or themes. For example - staff friendliness, staff professionalism, and staff efficiency can all be subtopics within the larger topic called Staff behavior. This allows the user to analyze the data at a broader level and at a granular level to identify the root cause.
Having granular topics also helps the user to future-proof the analysis to some extent, as there may be some topics about which customers may not be talking today but tomorrow when things change they may. For example – a website has been smoothly functioning, and there was no mention of bugs and crashes by customers in the past but due to a recent update more customers start talking about bugs and crashes. This insight gets picked up!
3. Platform capabilities
It is important to ascertain the nature of analysis the platform allows users to perform. Here is a list of a few key things to look out for.
Count/percentage of comments tagged to a topic
- Sentiment of the comments i.e., positive or negative. Alternately, having different topics for positive and negative experiences.
- Impact of each topic on KPI to help prioritize
- Ability to track and compare results across time and across different variables.
- Ability to understand the interplay between different topics to design an effective action plan.
- Predictive analysis, like understanding topics that are improving or deteriorating over time.
4. Visualizing the results
The interface of the platform determines the effectiveness and utility of the results. You may have the best technology to build topics, but if the user is not able to extract insights in an effective way, then it will only have a limited impact on the insights ecosystem of an organization.
This is an area in which even some of the big experience management platforms struggle as they have very limited visualizations for the text analytics outputs.
A few key features of an effective interface.
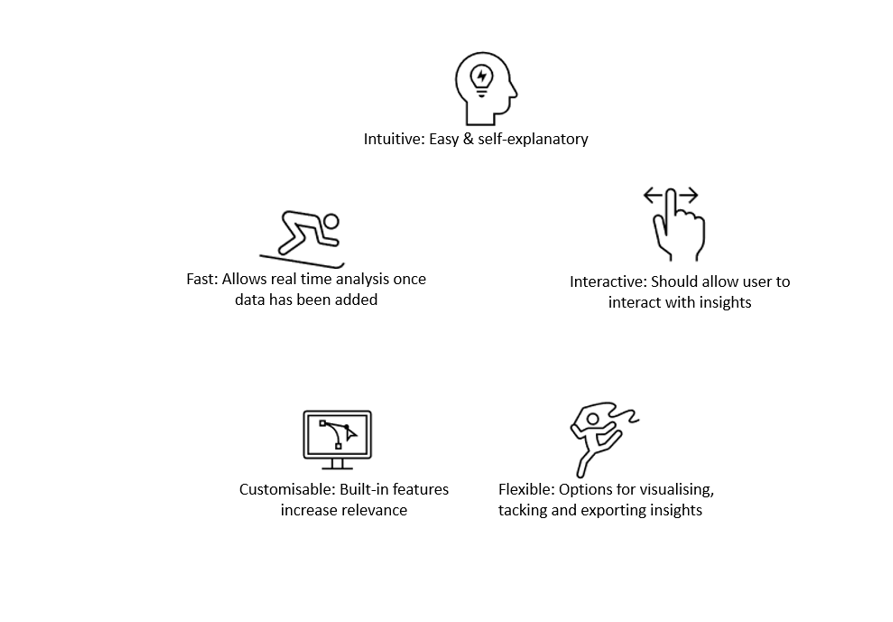
5. Onboarding and support from the provider
Text analytics is an evolving domain. Though it can open doors to an entirely new world of interesting insights, it can be quite overwhelming for those who are new to it. Be clear about what you are buying i.e. just access to the technology (platform) or technology + support / consulting services from the provider.
It is highly recommended that you start off with support from the provider to help you set up your analytics to your level of expectation, and then you may gradually reduce reliance on the provider as you get more comfortable with the platform. For example – you may need reporting support from the provider for the first 6 months before you take this responsibility upon yourself.
Internal champions with the right skillsets can be selected to take ownership, as building an understanding in this space requires time and patience.
General principles of text analytics
Users need to be mindful of the following general principles while working with text analytics.
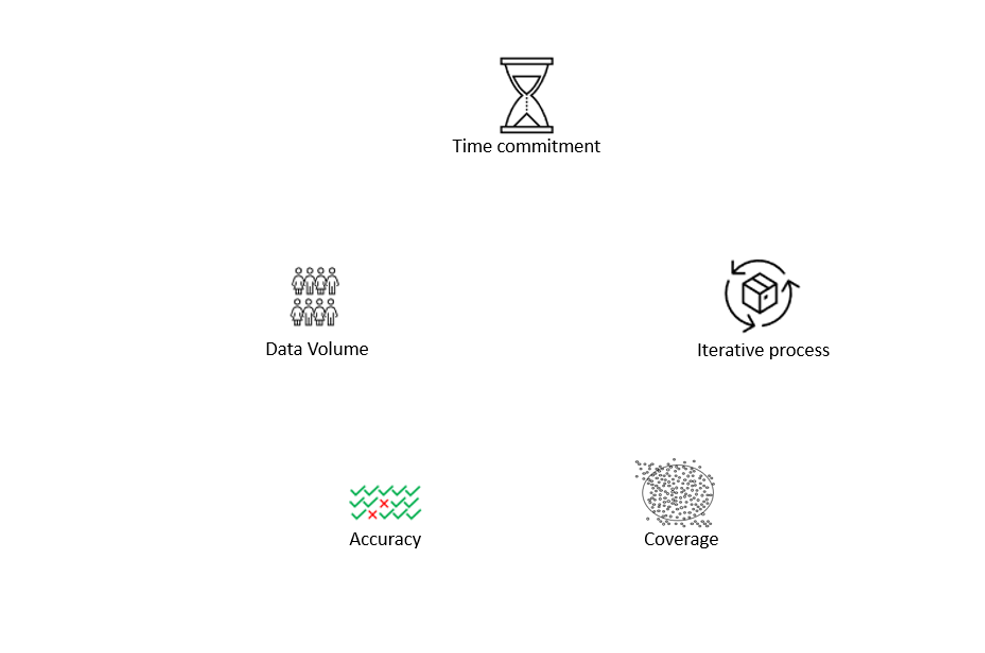
Time commitment
Though AI plays a key role in producing the results, we need to keep in mind it’s human-assisted AI, so you will need to be involved especially in the initial stages of setting up the topics or analyses. The results will only be as good as the setup.
Your time commitment will also depend to some extent on the nature of your agreement with the provider in terms of ownership and responsibility across different elements involved.
The iterative process
Once the topics/analyses have been set up to your satisfaction, you can start using the results. However, always be on the lookout to further sharpen the edge and spend some time going through the comments and the categorization to ensure you get what you need. Often, changes are also required based on feedback from other business stakeholders depending on their requirements. Of course, maximum effort will be required at the outset, and lesser effort will be required once the setup is complete.
Coverage
Coverage refers to the proportion of comments which have been tagged to any topic. Users need to systematically review unclassified comments to try and categorize them into different topics. The aim is to increase effective coverage by classifying comments across different topics of interest.
Accuracy
Accuracy is defined by the proportion of comments which have been correctly classified into their respective topics. There will be times when you may come across some text comments not being classified as expected. When you come across this, do not panic. Try to see if this can be fixed by altering your analysis setup. Text analytics is based on how people use words in their comments, and the text classification rules are designed to look at the right combination of words to categorize comments. Thus, there could be exceptions at times.
Don’t get into the trap of perfecting your analysis from an accuracy perspective before you start using it as you may never get there! However, certainly try and move closer to perfection. When in doubt, ask the question, does the big picture change? If you have done a good job with your set-up, the answer will mostly be ‘no’.
The volume of feedback to be analyzed
Text analytics works well with the higher volume of datasets. It makes sense to invest in text analytics if you have sizable data to analyze as the cost, time, and effort would be justified in that case. Tread with caution in case you are dealing with small datasets.
Conclusion
Advanced text analytics can take the quality of your insights to an entirely new level by empowering organizations with the information they need to make business decisions having a direct bearing on customer experience and, thus, the bottom line. A well-executed text analytics solution works like magic, but it does require time, effort, patience, and the right expertise to get it there.
About the author
Yazad Kakaria 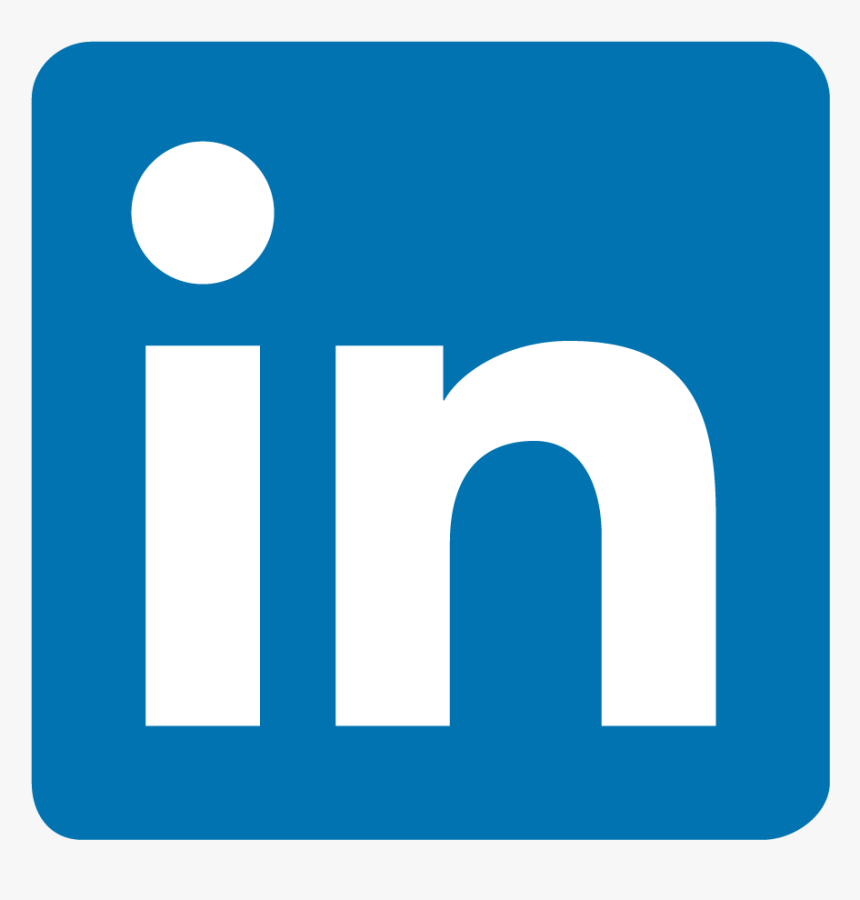
Account Director - Touchpoint Group
I have enjoyed working in the space of consumer insights & CX strategy with experience across different industries /sectors spanning agency and client side, as well as quantitative and qualitative research.
Having worked with clients across the globe addressing their insights needs by utilising some of the most advanced experience management platforms I have recently taken a plunge into the fascinating world of text analytics helping organisations uncover AI driven insights that lead to action planning.